Expo
view channel
view channel
view channel
view channel
view channel
Medical Imaging
AICritical CareSurgical TechniquesPatient CareHealth ITPoint of Care
Events
- Specialized Face Mask with Gas Sensor Detects Chronic Kidney Disease
- Implantable Device Continuously Monitors Brain Activity in Epileptic Patients
- Mechanosensing-Based Approach Offers Promising Strategy to Treat Cardiovascular Fibrosis
- AI Interpretability Tool for Photographed ECG Images Offers Pixel-Level Precision
- AI-ECG Tools Can Identify Heart Muscle Weakness in Women Before Pregnancy
- Bioprinted Aortas Offer New Hope for Vascular Repair
- Early TAVR Intervention Reduces Cardiovascular Events in Asymptomatic Aortic Stenosis Patients
- New Procedure Found Safe and Effective for Patients Undergoing Transcatheter Mitral Valve Replacement
- No-Touch Vein Harvesting Reduces Graft Failure Risk for Heart Bypass Patients
- DNA Origami Improves Imaging of Dense Pancreatic Tissue for Cancer Detection and Treatment
- First-Of-Its-Kind Portable Germicidal Light Technology Disinfects High-Touch Clinical Surfaces in Seconds
- Surgical Capacity Optimization Solution Helps Hospitals Boost OR Utilization
- Game-Changing Innovation in Surgical Instrument Sterilization Significantly Improves OR Throughput
- Next Gen ICU Bed to Help Address Complex Critical Care Needs
- Groundbreaking AI-Powered UV-C Disinfection Technology Redefines Infection Control Landscape
- Becton Dickinson to Spin Out Biosciences and Diagnostic Solutions Business
- Boston Scientific Acquires Medical Device Company SoniVie
- 2026 World Hospital Congress to be Held in Seoul
- Teleflex to Acquire BIOTRONIK’s Vascular Intervention Business
- Philips and Mass General Brigham Collaborate on Improving Patient Care with Live AI-Powered Insights
- Smartwatches Could Detect Congestive Heart Failure
- Versatile Smart Patch Combines Health Monitoring and Drug Delivery
- Machine Learning Model Improves Mortality Risk Prediction for Cardiac Surgery Patients
- Strategic Collaboration to Develop and Integrate Generative AI into Healthcare
- AI-Enabled Operating Rooms Solution Helps Hospitals Maximize Utilization and Unlock Capacity
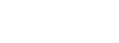
- Specialized Face Mask with Gas Sensor Detects Chronic Kidney Disease
- Implantable Device Continuously Monitors Brain Activity in Epileptic Patients
- Mechanosensing-Based Approach Offers Promising Strategy to Treat Cardiovascular Fibrosis
- AI Interpretability Tool for Photographed ECG Images Offers Pixel-Level Precision
- AI-ECG Tools Can Identify Heart Muscle Weakness in Women Before Pregnancy
- Bioprinted Aortas Offer New Hope for Vascular Repair
- Early TAVR Intervention Reduces Cardiovascular Events in Asymptomatic Aortic Stenosis Patients
- New Procedure Found Safe and Effective for Patients Undergoing Transcatheter Mitral Valve Replacement
- No-Touch Vein Harvesting Reduces Graft Failure Risk for Heart Bypass Patients
- DNA Origami Improves Imaging of Dense Pancreatic Tissue for Cancer Detection and Treatment
- First-Of-Its-Kind Portable Germicidal Light Technology Disinfects High-Touch Clinical Surfaces in Seconds
- Surgical Capacity Optimization Solution Helps Hospitals Boost OR Utilization
- Game-Changing Innovation in Surgical Instrument Sterilization Significantly Improves OR Throughput
- Next Gen ICU Bed to Help Address Complex Critical Care Needs
- Groundbreaking AI-Powered UV-C Disinfection Technology Redefines Infection Control Landscape
- Becton Dickinson to Spin Out Biosciences and Diagnostic Solutions Business
- Boston Scientific Acquires Medical Device Company SoniVie
- 2026 World Hospital Congress to be Held in Seoul
- Teleflex to Acquire BIOTRONIK’s Vascular Intervention Business
- Philips and Mass General Brigham Collaborate on Improving Patient Care with Live AI-Powered Insights
- Smartwatches Could Detect Congestive Heart Failure
- Versatile Smart Patch Combines Health Monitoring and Drug Delivery
- Machine Learning Model Improves Mortality Risk Prediction for Cardiac Surgery Patients
- Strategic Collaboration to Develop and Integrate Generative AI into Healthcare
- AI-Enabled Operating Rooms Solution Helps Hospitals Maximize Utilization and Unlock Capacity