Expo
view channel
view channel
view channel
view channel
view channel
Medical Imaging
Critical CareSurgical TechniquesPatient CareHealth ITPoint of CareBusiness
Events
- Heart Patch Offers Innovative Treatment Option for Advanced Heart Failure Patients
- Basal Cell Carcinoma Treatment Reduces Tumor Size, Improves Surgical Removal
- Light-Activated Ink Repairs Heart by Non-Invasively Manipulating Cardiac Tissue Activity
- New Epilepsy Tool Cuts Misdiagnoses by 70% Using Routine EEGs
- AI-Powered Prediction Model Enhances Blood Transfusion Decision-Making in ICU Patients
- Nanotechnology-Based Drug Delivery System Could Help Dialysis and Heart Patients Avoid Repeat Surgeries
- Groundbreaking Tool Accurately Predicts Stroke Outcome for Better Carotid Surgery Decisions
- Pioneering Technology Enables Real-Time Detection of Residual Breast Cancer During Lumpectomy
- Advanced Augmented Reality System to Transform Spine Surgery
- World's Smallest Multifunctional Biomedical Robot Holds Promise for Interventional Diagnosis and Treatment
- First-Of-Its-Kind Portable Germicidal Light Technology Disinfects High-Touch Clinical Surfaces in Seconds
- Surgical Capacity Optimization Solution Helps Hospitals Boost OR Utilization
- Game-Changing Innovation in Surgical Instrument Sterilization Significantly Improves OR Throughput
- Next Gen ICU Bed to Help Address Complex Critical Care Needs
- Groundbreaking AI-Powered UV-C Disinfection Technology Redefines Infection Control Landscape
- Boston Scientific Acquires Medical Device Company Intera Oncology
- MEDICA 2024 to Highlight Hot Topics of MedTech Industry
- Start-Ups To Once Again Play Starring Role at MEDICA 2024
- Boston Scientific to Acquire AFib Ablation Company Cortex
- Hologic Acquires Gynesonics to Strengthen Existing Gynecological Surgical Business
- Machine Learning Model Improves Mortality Risk Prediction for Cardiac Surgery Patients
- Strategic Collaboration to Develop and Integrate Generative AI into Healthcare
- AI-Enabled Operating Rooms Solution Helps Hospitals Maximize Utilization and Unlock Capacity
- AI Predicts Pancreatic Cancer Three Years before Diagnosis from Patients’ Medical Records
- First Fully Autonomous Generative AI Personalized Medical Authorizations System Reduces Care Delay
Expo
view channel
view channel
view channel
view channel
view channel
Medical Imaging
Critical CareSurgical TechniquesPatient CareHealth ITPoint of CareBusiness
Events
Advertise with Us
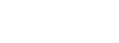
- Heart Patch Offers Innovative Treatment Option for Advanced Heart Failure Patients
- Basal Cell Carcinoma Treatment Reduces Tumor Size, Improves Surgical Removal
- Light-Activated Ink Repairs Heart by Non-Invasively Manipulating Cardiac Tissue Activity
- New Epilepsy Tool Cuts Misdiagnoses by 70% Using Routine EEGs
- AI-Powered Prediction Model Enhances Blood Transfusion Decision-Making in ICU Patients
- Nanotechnology-Based Drug Delivery System Could Help Dialysis and Heart Patients Avoid Repeat Surgeries
- Groundbreaking Tool Accurately Predicts Stroke Outcome for Better Carotid Surgery Decisions
- Pioneering Technology Enables Real-Time Detection of Residual Breast Cancer During Lumpectomy
- Advanced Augmented Reality System to Transform Spine Surgery
- World's Smallest Multifunctional Biomedical Robot Holds Promise for Interventional Diagnosis and Treatment
- First-Of-Its-Kind Portable Germicidal Light Technology Disinfects High-Touch Clinical Surfaces in Seconds
- Surgical Capacity Optimization Solution Helps Hospitals Boost OR Utilization
- Game-Changing Innovation in Surgical Instrument Sterilization Significantly Improves OR Throughput
- Next Gen ICU Bed to Help Address Complex Critical Care Needs
- Groundbreaking AI-Powered UV-C Disinfection Technology Redefines Infection Control Landscape
- Boston Scientific Acquires Medical Device Company Intera Oncology
- MEDICA 2024 to Highlight Hot Topics of MedTech Industry
- Start-Ups To Once Again Play Starring Role at MEDICA 2024
- Boston Scientific to Acquire AFib Ablation Company Cortex
- Hologic Acquires Gynesonics to Strengthen Existing Gynecological Surgical Business
- Machine Learning Model Improves Mortality Risk Prediction for Cardiac Surgery Patients
- Strategic Collaboration to Develop and Integrate Generative AI into Healthcare
- AI-Enabled Operating Rooms Solution Helps Hospitals Maximize Utilization and Unlock Capacity
- AI Predicts Pancreatic Cancer Three Years before Diagnosis from Patients’ Medical Records
- First Fully Autonomous Generative AI Personalized Medical Authorizations System Reduces Care Delay