Expo
view channel
view channel
view channel
view channel
Medical Imaging
AI
Surgical TechniquesPatient CareHealth ITPoint of CareBusiness
Events
Webinars
- First Of Its Kind Adhesive Offers More Comfortable Alternative for Wearable Medical Devices
- Discovery of Heart’s Hidden Geometry to Revolutionize ECG Interpretation
- New Approach Improves Diagnostic Accuracy for Esophageal Motility Disorders
- Wristband Sensor Provides All-In-One Monitoring for Diabetes and Cardiovascular Care
- Handheld Device Enables Imaging and Treatment of Oral Cancer in Low-Resource Settings
- 3D Printed Implant to Help Repair Spinal Cord Injuries
- Fluorescent Imaging Agent ‘Lights Up’ Nerves for Better Visualization During Surgery
- LED-Based Imaging System Could Transform Cancer Detection in Endoscopy
- New Surgical Microscope Offers Precise 3D Imaging Using 48 Tiny Cameras
- First-Of-Its-Kind Drug Illuminates Nerve Tissue for Faster and Safer Surgery
- VR Training Tool Combats Contamination of Portable Medical Equipment
- Portable Biosensor Platform to Reduce Hospital-Acquired Infections
- First-Of-Its-Kind Portable Germicidal Light Technology Disinfects High-Touch Clinical Surfaces in Seconds
- Surgical Capacity Optimization Solution Helps Hospitals Boost OR Utilization
- Game-Changing Innovation in Surgical Instrument Sterilization Significantly Improves OR Throughput
- Medtronic Partners with Corsano to Expand Acute Care & Monitoring Portfolio in Europe
- Expanded Collaboration to Transform OR Technology Through AI and Automation
- Becton Dickinson to Spin Out Biosciences and Diagnostic Solutions Business
- Boston Scientific Acquires Medical Device Company SoniVie
- 2026 World Hospital Congress to be Held in Seoul
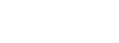
- First Of Its Kind Adhesive Offers More Comfortable Alternative for Wearable Medical Devices
- Discovery of Heart’s Hidden Geometry to Revolutionize ECG Interpretation
- New Approach Improves Diagnostic Accuracy for Esophageal Motility Disorders
- Wristband Sensor Provides All-In-One Monitoring for Diabetes and Cardiovascular Care
- Handheld Device Enables Imaging and Treatment of Oral Cancer in Low-Resource Settings
- 3D Printed Implant to Help Repair Spinal Cord Injuries
- Fluorescent Imaging Agent ‘Lights Up’ Nerves for Better Visualization During Surgery
- LED-Based Imaging System Could Transform Cancer Detection in Endoscopy
- New Surgical Microscope Offers Precise 3D Imaging Using 48 Tiny Cameras
- First-Of-Its-Kind Drug Illuminates Nerve Tissue for Faster and Safer Surgery
- VR Training Tool Combats Contamination of Portable Medical Equipment
- Portable Biosensor Platform to Reduce Hospital-Acquired Infections
- First-Of-Its-Kind Portable Germicidal Light Technology Disinfects High-Touch Clinical Surfaces in Seconds
- Surgical Capacity Optimization Solution Helps Hospitals Boost OR Utilization
- Game-Changing Innovation in Surgical Instrument Sterilization Significantly Improves OR Throughput
- Medtronic Partners with Corsano to Expand Acute Care & Monitoring Portfolio in Europe
- Expanded Collaboration to Transform OR Technology Through AI and Automation
- Becton Dickinson to Spin Out Biosciences and Diagnostic Solutions Business
- Boston Scientific Acquires Medical Device Company SoniVie
- 2026 World Hospital Congress to be Held in Seoul